Uncovering the Communities with the Highest Premature Deaths in Ontario
August 7/2019
U of T researchers have conducted the first spatial analysis of death in Ontario, discovering that social and demographic factors are by far the biggest factors in predicting who dies before their time.
Public health researchers used traditional statistical and geography tools to break down premature death rates community by community in Ontario, allowing them to pinpoint clusters with the highest numbers of people dying before age 75. They found sizeable disparities in premature mortality, with the majority of premature deaths occurring in low-income neighbourhoods and the fewest in immigrant communities.
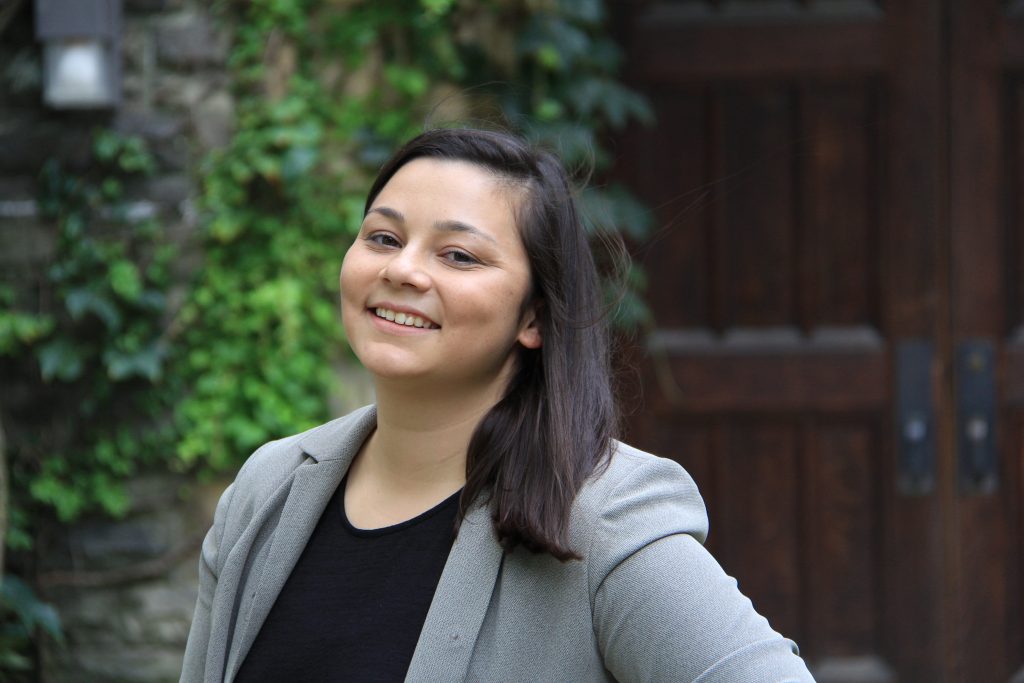
Alumnus Emmalin Buajitti
“The fact that immigrant and socio-economic status alone accounted for 80 percent of the variation – that’s a staggering amount,” says the study’s co-author, Emmalin Buajitti, a recent graduate of the Master’s of Public Health program at the Dalla Lana School of Public Health. “This emphasizes the enormous importance of ‘social determinants’ of health.”
Only 10 percent of the variation was further explained by classic risks like heavy drinking, obesity, smoking and sedentary lifestyles, and another 10 percent was unexplained, but possibly due to other factors such as lack of access to medical care, Buajitti says. While there would be crossover between risk factors and poverty, she says, income and was still a far more powerful predictor of premature death than lifestyle alone. And lower premature mortality among immigrants is not surprising given that Canada chooses people in good health to migrate here.
The most common cause of premature deaths, not surprisingly, was heart disease – the same as for non-premature deaths in Ontario. Men died early at higher rates than women, as did people in more northern, rural and underserved parts of the province. The Toronto and Ottawa metropolitan areas had the lowest rates of premature mortality.
To identify death patterns on a granular level, Buajitti and her co-authors, including Big Data expert and DLSPH Prof. Laura C. Rosella, divided the province’s local health regions into 76 sub-regions. They used Bayesian models to map early deaths to known risk factors such as smoking and poverty.
“The techniques we used aren’t that new – they’ve been established, especially among geographers, for some time,” says Buajitti. “The opportunities come from bridging disciplinary gaps – using geography to answer better public health questions, and identify patterns more precisely. In Canada, these techniques haven’t been applied on this scale.”
The geography of health is an important area to study, she says, because there’s a connection between a person’s health and the overall health of the population in their community: “You can be doing quite well personally, not smoking or drinking heavily, but your neighbourhood might not have enough doctors or parks and sidewalks, or it might be a food desert,” she says. “We’re trying to answer the impact your neighbourhood experience has on health and premature mortality so that public health interventions can be targeted where they’re needed most.”
Buajitti and her co-authors published the results of their research Aug.1 in the journal Population Health Metrics.