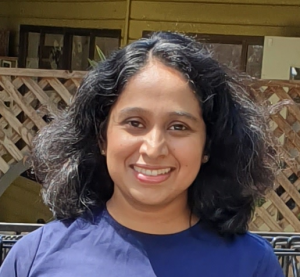
Kanchana Wickramasinghe
Griffith University, Australia
kanchana.wickramasinghe@gmail.com
Food systems have become more complex due to increasing urbanization, income growth and globalization1. Further, the COVID-19 pandemic has led to increase vulnerabilities on food systems and associated components such as food production and food insecurity among vulnerable communities. Comprehensive understanding of potential co-benefits is important in generating multiple benefits from post-COVID policy interventions. The proposed research intends to map the connections between important components of food systems, health and environment systems and their outcomes using a quantitative approach.
Studies point out the need for policy level changes to reduce the premature NCD mortality in Sri Lanka, which has been showing a notable increase2. Specific behavioural risk factors such as physical activities, dietary habits, smoking, alcohol consumption and obesity3 and urbanicity4 have found to have significant impacts on prevalence of NCDs. Further Sri Lanka is identified as a highly vulnerable country to the impacts of climate change and agriculture sector is particularly vulnerable climate impacts5.
Alignment of co-benefits at the intersection of Health and Environments
The proposed study will focus on health outcomes at the intersection of food systems, environment (including climate change) and household economics using data from Sri Lanka. The health dimension will be mainly addressed via the NCDs which have a strong relationship with several facets in the food systems in low- and middle-income countries. The impacts due to NCDs are particularly high in South Asia6. Other diseases will also be considered depending on their significance in the interlinkages between health, environment, and food systems.
More specifically, the study will attempt to categorize the households based on health risks and to predict such risks using variables that cover the dimensions of food systems (such as production and consumption), environmental conditions, and climate change vulnerabilities. The study will deviate from commonly available descriptive approaches to produce quantitative estimates by accommodating individual, household and district level data from Sri Lanka. Our aim goes beyond the objective of the econometric approaches which aim at estimation of parameters that underlie the relationship between dependent and independent variables. From a policy perspective, it is highly important to have empirical information on risk prediction in relation to health, food systems and environmental interlinkages.
Relevant conceptual theories, methodological tools, frameworks, approaches, gender and equity considerations
Spotlight on references:
Brouwer ID, et al. Food systems everywhere: Improving relevance in practice. Global Food Security 2020;26,100398.
Parsons K, Hawkes C. Connecting Food Systems for Co-Benefits: How Can Food Systems Combine Diet-Related Health with Environmental and Economic Policy Goals? Policy Brief 31; Denmark: World Health Organisation (WHO Regional Office for Europe) 2018.
- Initial analysis of the study primarily based on the conceptual framework presented in Brouwer et al.7 adapted from HLPE8 and the “A vision for food systems with co-benefits” framework developed by Parsons & Hawkes9. These frameworks provide the basis for identifying specific variables and indicators for the quantitative assessment.
- Machine learning (ML) approach for predicting health risks based on the food system and environmental dimensions using individual, household and district level data from Sri Lanka. ML is a versatile approach that has direct policy applications10.
We will employ supervised ML techniques to understand the linkages between the food systems, health outcomes, environmental factors, and climate change in Sri Lanka. The analysis will involve classification of households to several categories, based on the prevalence of NCDs among the household members. A household level index will be developed based on prevalence of NCDs. The index is used in clustering the households based on NCD prevalence. The next step will involve feeding the socio-economic, demographic, food-system related aspects and environmental factors into the model and assess how accurately the model can classify the households into different categories based on NCD prevalence. The model will be then trained to get an accurate prediction, so that it can be used to identify the vulnerable households. Interestingly, the model will also be used to detect the potential households who are at a risk.
The quantitative analysis helps in understanding the significant interlinkages between health, environment, and food systems at household level. The interlinkages can be used in identifying the potential avenues for creating co-benefits through policy interventions. More specifically, the findings of the study will be helpful in addressing the issues in health outcomes in terms of food system and environmental changes.
The proposed approach provides the flexibility to carry out the analysis for different segments of population. It is intended to use data from the Household Income and Expenditure Survey conducted by the Department of Census and Statistics of Sri Lanka. The survey is carried out usually once in three years and the study will use data from the latest round of survey conducted in 2018. The Survey identifies the gender, education status, age, health outcomes, income, etc. at individual level. This will provide an opportunity to understand the gender-based differences regarding health outcomes. In addition, the analysis can potentially be extended to perform detailed analysis based on other socio-economic groups. The supervised ML models will be first trained using the historical dataset using pre-identified target variable and the features. An accurate model which can predict the target reasonably well will be identified by employing rigorous validation techniques.
Available techniques that could reduce overfitting of ML models will be also incorporated into modelling. Moreover, qualitative variables such as gender and other social or political variables can be used as inputs in these models, thus we could minimise any biases that could creep into the outcome. Furthermore, we are planning to adopt a range of ML algorithms, particularly the deep learning models, so that any inherent biases exist in the algorithms can be minimised.
These socio-economic groups may be based on individual/household income (poverty groups), employment categories, occupation types, sectors (rural and urban), districts, agricultural dependence, etc. Such detailed analysis will help in understanding the differential behaviours of such groups at the interlinkages between health, environment, and food systems. Our quantitative findings will thus provide an indication about the level of potential co-benefits among the identified groups.
Measurement of impact
Spotlight on references:
Mayrhofer JP, Gupta J. The science and politics of co-benefits in climate policy. Environmental Science & Policy 2016;57,22-30.
Cohen B, et al. Co-benefits and trade-offs of climate change mitigation actions and the Sustainable Development Goals. Sustainable Production and Consumption 2021;26,805-813.
The derivation of specific indicators for measuring the co-benefits will be based on the significant inter-linkages that will be identified through ML analysis. Potential indicators may include:
- Disease prevalence by gender, socio-economic (income, employment etc.), sector (rural and urban), geographic, and other important categories;
- Proportion of sustainable food production incorporating the aspects of chemical usage, subsistence food production;
- Environmental outcomes and climate adaptation indicators including natural disaster impacts, housing characteristics, dependence on natural resources (land, forests, fisheries), household energy consumption;
- Socio-economic outcomes including level of employment, income diversification, education level, indebtedness
Interestingly, the idea of co-benefits includes political weight in its definition, application and use11. As highlighted by Cohen et al.12, the co-benefits approach has the potential to encourage collaborative action of stakeholders with regard to climate change interventions. This aspect can be effectively extended in influencing the stakeholders regarding promoting health and environmental co-benefits regarding food systems. The findings of the study will be mainly used to influence policy makers in an array of development areas including health and nutrition, agriculture, climate change, natural resource management, environment, and social welfare. The findings will also be useful for development agencies, non-governmental organizations, and donors in assessing the potential impacts of their development interventions.
Challenges/barriers and knowledge gaps impeding shifts towards healthier and sustainable food systems
Spotlight on references:
Berrang-Ford L, et al. Systematic mapping of global research on climate and health: a machine learning review. The Lancet Planetary Health 2021;5(8),e514-e525.
Athey S. The impact of machine learning on economics. In Agrawal A, Gans J, Goldfarb A, editors. The economics of artificial intelligence: An agenda. US:University of Chicago Press; 2018.p. 507-547.
Storm H, et al. Machine learning in agricultural and applied economics. European Review of Agricultural Economics 2020; 47(3),849-892.
The idea of co-benefits has been receiving an increasing attention in previous work that aims at reconciling environment and developmental goals13. Our review of literature regarding health, environment and food systems indicates that existing studies mostly are qualitative in nature. This creates challenges in understanding the comparative magnitudes of the co-benefits created by specific policy interventions. Further, a review done by Berrang-Ford et al.14 find that the existing literature that focus on climate change and health has paid little attention towards solutions and co-benefits of policy interventions. Brouwer et al.15 points out that the critical trade-offs in food systems and required policy interventions are not sufficiently studied in the literature.
Regarding policy interventions, lack of collaborative efforts among the policy makers and other stakeholders has been a key obstacle in realizing co-benefits. This may be particularly true for low- and middle-income countries and Sri Lanka is no exception.
We intend to apply ML approaches to perform a predictive analysis, which is a novel and growing field in agricultural and applied economics16. Data-driven model selection is a common characteristic of many ML methods. This feature allows development of algorithm that can examine several alternatives and then select the best model17. Such data-driven modelling techniques allow producing interdisciplinary and holistic solutions to complex issues including socio-ecological issues18.
A common limitation in ML and other statistical/econometric models is that they are unable to capture some qualitative features that are driving the target variable. Also, there can be overfitting issues in ML models and this can be controlled to some extent by adopting recently developed techniques. As highlighted previously, unlike in econometric models, ML models cannot explicitly estimate responsiveness of different variables in causality analysis. However, in terms of prediction power, ML models are much superior to other standard models. The proposed study also will present a methodological contribution to the literature using ML approaches, by illustrating how household level data can be utilized to understand the complex relationships in food systems and design necessary policy interventions.
References
- ^ Fanzo J. Healthy and sustainable diets and food systems: the key to achieving Sustainable Development Goal 2? Food ethics 2019;4(2):159-174.
- ^ Ediriweera DS, Karunapema P, Pathmeswaran A, Arnold M. Increase in premature mortality due to non-communicable diseases in Sri Lanka during the first decade of the twenty-first century. BMC public health 2018;18(1):584.
- ^ Gamage AU, Jayawardana PL. Knowledge of non-communicable diseases and practices related to healthy lifestyles among adolescents, in state schools of a selected educational division in Sri Lanka. BMC public health 2017;8(1):64.
- ^ Allender S, Wickramasinghe K, Goldacre M, Matthews D, Katulanda P. Quantifying urbanization as a risk factor for noncommunicable disease. Journal of urban health 2011;88(5):906-18.
- ^ Wickramasinghe K. Climate Insurance for Dry Zone Farmers in Sri Lanka: Prospects for Index Insurance. Agricultural research series no. 200, Sri Lanka; Institute of Policy Studies of Sri Lanka. 2019.
- ^ Ghaffar A, Reddy KS, Singhi M. Burden of non-communicable diseases in South Asia. British Medical Journal 2004;328(7443):807-810.
- ^ Brouwer ID, McDermott J, Ruben R. Food systems everywhere: Improving relevance in practice. Global Food Security 2020;26:100398.
- ^ HLPE. Nutrition and food systems. A report by the High Level Panel of Experts on Food Security and Nutrition of the Committee on World Food Security, Rome: 2018.
- ^ Parsons K, Hawkes C. Connecting Food Systems for Co-Benefits: How Can Food Systems Combine Diet-Related Health with Environmental and Economic Policy Goals? Policy Brief 31; Denmark: World Health Organisation (WHO Regional Office for Europe) 2018.
- ^ Mullainathan S, Spiess J. Machine Learning: An applied econometric approach. Journal of Economic Perspectives; 2017:31(2): 87–106
- ^ Mayrhofer JP, Gupta J. The science and politics of co-benefits in climate policy. Environmental Science & Policy 2016;57:22-30.
- ^ Cohen B, Cowie A, Babiker M, Leip A, Smith P. Co-benefits and trade-offs of climate change mitigation actions and the Sustainable Development Goals. Sustainable Production and Consumption 2021; 26:805-813.
- ^ Mayrhofer JP, Gupta J. The science and politics of co-benefits in climate policy. Environmental Science & Policy 2016;57:22-30.
- ^ Berrang-Ford L, Sietsma AJ, Callaghan M, Minx JC, Scheelbeek PF, Haddaway NR, Haines A, Dangour AD. Systematic mapping of global research on climate and health: a machine learning review. The Lancet Planetary Health 2021;5(8):e514-e525.
- ^ Brouwer ID, McDermott J, Ruben R. Food systems everywhere: Improving relevance in practice. Global Food Security 2020;26:100398.
- ^ Storm H, Baylis K, Heckelei T. Machine learning in agricultural and applied economics. European Review of Agricultural Economics 2020;47(3):849-892.
- ^ Athey S. The impact of machine learning on economics. In Agrawal A, Gans J, Goldfarb A, editors. The economics of artificial intelligence: An agenda. US:University of Chicago Press; 2018;p.507-547.
- ^ Willcock S, Martínez-López J, Hooftman DA, Bagstad KJ, Balbi S, Marzo A, Prato C, Sciandrello S, Signorello G, Voigt B. Machine learning for ecosystem services. Ecosystem services 2018;33:165-174.